Why Most Published Research Findings Are False
Summary
There is increasing concern that most current published research findings are false. The probability that a research claim is true may depend on study power and bias, the number of other studies on the same question, and, importantly, the ratio of true to no relationships among the relationships probed in each scientific field. In this framework, a research finding is less likely to be true when the studies conducted in a field are smaller; when effect sizes are smaller; when there is a greater number and lesser preselection of tested relationships; where there is greater flexibility in designs, definitions, outcomes, and analytical modes; when there is greater financial and other interest and prejudice; and when more teams are involved in a scientific field in chase of statistical significance. Simulations show that for most study designs and settings, it is more likely for a research claim to be false than true. Moreover, for many current scientific fields, claimed research findings may often be simply accurate measures of the prevailing bias. In this essay, I discuss the implications of these problems for the conduct and interpretation of research.
Published research findings are sometimes refuted by subsequent evidence, with ensuing confusion and disappointment. Refutation and controversy is seen across the range of research designs, from clinical trials and traditional epidemiological studies [1–3] to the most modern molecular research [4,5]. There is increasing concern that in modern research, false findings may be the majority or even the vast majority of published research claims [6–8]. However, this should not be surprising. It can be proven that most claimed research findings are false. Here I will examine the key factors that influence this problem and some corollaries thereof.
Modeling the Framework for False Positive Findings
Several methodologists have pointed out [9–11] that the high rate of nonreplication (lack of confirmation) of research discoveries is a consequence of the convenient, yet ill-founded strategy of claiming conclusive research findings solely on the basis of a single study assessed by formal statistical significance, typically for a p-value less than 0.05. Research is not most appropriately represented and summarized by p-values, but, unfortunately, there is a widespread notion that medical research articles should be interpreted based only on p-values. Research findings are defined here as any relationship reaching formal statistical significance, e.g., effective interventions, informative predictors, risk factors, or associations. “Negative” research is also very useful. “Negative” is actually a misnomer, and the misinterpretation is widespread. However, here we will target relationships that investigators claim exist, rather than null findings.
It can be proven that most claimed research findings are false
As has been shown previously, the probability that a research finding is indeed true depends on the prior probability of it being true (before doing the study), the statistical power of the study, and the level of statistical significance [10,11]. Consider a 2 × 2 table in which research findings are compared against the gold standard of true relationships in a scientific field. In a research field both true and false hypotheses can be made about the presence of relationships. Let R be the ratio of the number of “true relationships” to “no relationships” among those tested in the field. R is characteristic of the field and can vary a lot depending on whether the field targets highly likely relationships or searches for only one or a few true relationships among thousands and millions of hypotheses that may be postulated. Let us also consider, for computational simplicity, circumscribed fields where either there is only one true relationship (among many that can be hypothesized) or the power is similar to find any of the several existing true relationships. The pre-study probability of a relationship being true is R/(R + 1). The probability of a study finding a true relationship reflects the power 1 – β (one minus the Type II error rate). The probability of claiming a relationship when none truly exists reflects the Type I error rate, α. Assuming that c relationships are being probed in the field, the expected values of the 2 × 2 table are given in . After a research finding has been claimed based on achieving formal statistical significance, the post-study probability that it is true is the positive predictive value, PPV. The PPV is also the complementary probability of what Wacholder et al. have called the false positive report probability [10]. According to the 2 × 2 table, one gets PPV = (1 – β)R/(R – βR + α). A research finding is thus more likely true than false if (1 – β)R > α. Since usually the vast majority of investigators depend on a = 0.05, this means that a research finding is more likely true than false if (1 – β)R > 0.05.
Table 1
Research Findings and True Relationships
What is less well appreciated is that bias and the extent of repeated independent testing by different teams of investigators around the globe may further distort this picture and may lead to even smaller probabilities of the research findings being indeed true. We will try to model these two factors in the context of similar 2 × 2 tables.
Bias
First, let us define bias as the combination of various design, data, analysis, and presentation factors that tend to produce research findings when they should not be produced. Let u be the proportion of probed analyses that would not have been “research findings,” but nevertheless end up presented and reported as such, because of bias. Bias should not be confused with chance variability that causes some findings to be false by chance even though the study design, data, analysis, and presentation are perfect. Bias can entail manipulation in the analysis or reporting of findings. Selective or distorted reporting is a typical form of such bias. We may assume that u does not depend on whether a true relationship exists or not. This is not an unreasonable assumption, since typically it is impossible to know which relationships are indeed true. In the presence of bias (), one gets PPV = ([1 – β]R + uβR)/(R + α − βR + u − uα + uβR), and PPV decreases with increasing u, unless 1 − β ≤ α, i.e., 1 − β ≤ 0.05 for most situations. Thus, with increasing bias, the chances that a research finding is true diminish considerably. This is shown for different levels of power and for different pre-study odds in . Conversely, true research findings may occasionally be annulled because of reverse bias. For example, with large measurement errors relationships are lost in noise [12], or investigators use data inefficiently or fail to notice statistically significant relationships, or there may be conflicts of interest that tend to “bury” significant findings [13]. There is no good large-scale empirical evidence on how frequently such reverse bias may occur across diverse research fields. However, it is probably fair to say that reverse bias is not as common. Moreover measurement errors and inefficient use of data are probably becoming less frequent problems, since measurement error has decreased with technological advances in the molecular era and investigators are becoming increasingly sophisticated about their data. Regardless, reverse bias may be modeled in the same way as bias above. Also reverse bias should not be confused with chance variability that may lead to missing a true relationship because of chance.
PPV (Probability That a Research Finding Is True) as a Function of the Pre-Study Odds for Various Levels of Bias, uPanels correspond to power of 0.20, 0.50, and 0.80.
Table 2
Research Findings and True Relationships in the Presence of Bias
Testing by Several Independent Teams
Several independent teams may be addressing the same sets of research questions. As research efforts are globalized, it is practically the rule that several research teams, often dozens of them, may probe the same or similar questions. Unfortunately, in some areas, the prevailing mentality until now has been to focus on isolated discoveries by single teams and interpret research experiments in isolation. An increasing number of questions have at least one study claiming a research finding, and this receives unilateral attention. The probability that at least one study, among several done on the same question, claims a statistically significant research finding is easy to estimate. For n independent studies of equal power, the 2 × 2 table is shown in : PPV = R(1 − βn)/(R + 1 − [1 − α]n − Rβn) (not considering bias). With increasing number of independent studies, PPV tends to decrease, unless 1 – β < a, i.e., typically 1 − β < 0.05. This is shown for different levels of power and for different pre-study odds in . For n studies of different power, the term βn is replaced by the product of the terms βi for i = 1 to n, but inferences are similar.
PPV (Probability That a Research Finding Is True) as a Function of the Pre-Study Odds for Various Numbers of Conducted Studies, nPanels correspond to power of 0.20, 0.50, and 0.80.
Table 3
Research Findings and True Relationships in the Presence of Multiple Studies
Corollaries
A practical example is shown in Box 1. Based on the above considerations, one may deduce several interesting corollaries about the probability that a research finding is indeed true.
Box 1. An Example: Science at Low Pre-Study Odds
Let us assume that a team of investigators performs a whole genome association study to test whether any of 100,000 gene polymorphisms are associated with susceptibility to schizophrenia. Based on what we know about the extent of heritability of the disease, it is reasonable to expect that probably around ten gene polymorphisms among those tested would be truly associated with schizophrenia, with relatively similar odds ratios around 1.3 for the ten or so polymorphisms and with a fairly similar power to identify any of them. Then R = 10/100,000 = 10−4, and the pre-study probability for any polymorphism to be associated with schizophrenia is also R/(R + 1) = 10−4. Let us also suppose that the study has 60% power to find an association with an odds ratio of 1.3 at α = 0.05. Then it can be estimated that if a statistically significant association is found with the p-value barely crossing the 0.05 threshold, the post-study probability that this is true increases about 12-fold compared with the pre-study probability, but it is still only 12 × 10−4.
Now let us suppose that the investigators manipulate their design, analyses, and reporting so as to make more relationships cross the p = 0.05 threshold even though this would not have been crossed with a perfectly adhered to design and analysis and with perfect comprehensive reporting of the results, strictly according to the original study plan. Such manipulation could be done, for example, with serendipitous inclusion or exclusion of certain patients or controls, post hoc subgroup analyses, investigation of genetic contrasts that were not originally specified, changes in the disease or control definitions, and various combinations of selective or distorted reporting of the results. Commercially available “data mining” packages actually are proud of their ability to yield statistically significant results through data dredging. In the presence of bias with u = 0.10, the post-study probability that a research finding is true is only 4.4 × 10−4. Furthermore, even in the absence of any bias, when ten independent research teams perform similar experiments around the world, if one of them finds a formally statistically significant association, the probability that the research finding is true is only 1.5 × 10−4, hardly any higher than the probability we had before any of this extensive research was undertaken!
Corollary 1: The smaller the studies conducted in a scientific field, the less likely the research findings are to be true. Small sample size means smaller power and, for all functions above, the PPV for a true research finding decreases as power decreases towards 1 − β = 0.05. Thus, other factors being equal, research findings are more likely true in scientific fields that undertake large studies, such as randomized controlled trials in cardiology (several thousand subjects randomized) [14] than in scientific fields with small studies, such as most research of molecular predictors (sample sizes 100-fold smaller) [15].
Corollary 2: The smaller the effect sizes in a scientific field, the less likely the research findings are to be true. Power is also related to the effect size. Thus research findings are more likely true in scientific fields with large effects, such as the impact of smoking on cancer or cardiovascular disease (relative risks 3–20), than in scientific fields where postulated effects are small, such as genetic risk factors for multigenetic diseases (relative risks 1.1–1.5) [7]. Modern epidemiology is increasingly obliged to target smaller effect sizes [16]. Consequently, the proportion of true research findings is expected to decrease. In the same line of thinking, if the true effect sizes are very small in a scientific field, this field is likely to be plagued by almost ubiquitous false positive claims. For example, if the majority of true genetic or nutritional determinants of complex diseases confer relative risks less than 1.05, genetic or nutritional epidemiology would be largely utopian endeavors.
Corollary 3: The greater the number and the lesser the selection of tested relationships in a scientific field, the less likely the research findings are to be true. As shown above, the post-study probability that a finding is true (PPV) depends a lot on the pre-study odds (R). Thus, research findings are more likely true in confirmatory designs, such as large phase III randomized controlled trials, or meta-analyses thereof, than in hypothesis-generating experiments. Fields considered highly informative and creative given the wealth of the assembled and tested information, such as microarrays and other high-throughput discovery-oriented research [4,8,17], should have extremely low PPV.
Corollary 4: The greater the flexibility in designs, definitions, outcomes, and analytical modes in a scientific field, the less likely the research findings are to be true. Flexibility increases the potential for transforming what would be “negative” results into “positive” results, i.e., bias, u. For several research designs, e.g., randomized controlled trials [18–20] or meta-analyses [21,22], there have been efforts to standardize their conduct and reporting. Adherence to common standards is likely to increase the proportion of true findings. The same applies to outcomes. True findings may be more common when outcomes are unequivocal and universally agreed (e.g., death) rather than when multifarious outcomes are devised (e.g., scales for schizophrenia outcomes) [23]. Similarly, fields that use commonly agreed, stereotyped analytical methods (e.g., Kaplan-Meier plots and the log-rank test) [24] may yield a larger proportion of true findings than fields where analytical methods are still under experimentation (e.g., artificial intelligence methods) and only “best” results are reported. Regardless, even in the most stringent research designs, bias seems to be a major problem. For example, there is strong evidence that selective outcome reporting, with manipulation of the outcomes and analyses reported, is a common problem even for randomized trails [25]. Simply abolishing selective publication would not make this problem go away.
Corollary 5: The greater the financial and other interests and prejudices in a scientific field, the less likely the research findings are to be true. Conflicts of interest and prejudice may increase bias, u. Conflicts of interest are very common in biomedical research [26], and typically they are inadequately and sparsely reported [26,27]. Prejudice may not necessarily have financial roots. Scientists in a given field may be prejudiced purely because of their belief in a scientific theory or commitment to their own findings. Many otherwise seemingly independent, university-based studies may be conducted for no other reason than to give physicians and researchers qualifications for promotion or tenure. Such nonfinancial conflicts may also lead to distorted reported results and interpretations. Prestigious investigators may suppress via the peer review process the appearance and dissemination of findings that refute their findings, thus condemning their field to perpetuate false dogma. Empirical evidence on expert opinion shows that it is extremely unreliable [28].
Corollary 6: The hotter a scientific field (with more scientific teams involved), the less likely the research findings are to be true. This seemingly paradoxical corollary follows because, as stated above, the PPV of isolated findings decreases when many teams of investigators are involved in the same field. This may explain why we occasionally see major excitement followed rapidly by severe disappointments in fields that draw wide attention. With many teams working on the same field and with massive experimental data being produced, timing is of the essence in beating competition. Thus, each team may prioritize on pursuing and disseminating its most impressive “positive” results. “Negative” results may become attractive for dissemination only if some other team has found a “positive” association on the same question. In that case, it may be attractive to refute a claim made in some prestigious journal. The term Proteus phenomenon has been coined to describe this phenomenon of rapidly alternating extreme research claims and extremely opposite refutations [29]. Empirical evidence suggests that this sequence of extreme opposites is very common in molecular genetics [29].
These corollaries consider each factor separately, but these factors often influence each other. For example, investigators working in fields where true effect sizes are perceived to be small may be more likely to perform large studies than investigators working in fields where true effect sizes are perceived to be large. Or prejudice may prevail in a hot scientific field, further undermining the predictive value of its research findings. Highly prejudiced stakeholders may even create a barrier that aborts efforts at obtaining and disseminating opposing results. Conversely, the fact that a field is hot or has strong invested interests may sometimes promote larger studies and improved standards of research, enhancing the predictive value of its research findings. Or massive discovery-oriented testing may result in such a large yield of significant relationships that investigators have enough to report and search further and thus refrain from data dredging and manipulation.
Most Research Findings Are False for Most Research Designs and for Most Fields
In the described framework, a PPV exceeding 50% is quite difficult to get. provides the results of simulations using the formulas developed for the influence of power, ratio of true to non-true relationships, and bias, for various types of situations that may be characteristic of specific study designs and settings. A finding from a well-conducted, adequately powered randomized controlled trial starting with a 50% pre-study chance that the intervention is effective is eventually true about 85% of the time. A fairly similar performance is expected of a confirmatory meta-analysis of good-quality randomized trials: potential bias probably increases, but power and pre-test chances are higher compared to a single randomized trial. Conversely, a meta-analytic finding from inconclusive studies where pooling is used to “correct” the low power of single studies, is probably false if R ≤ 1:3. Research findings from underpowered, early-phase clinical trials would be true about one in four times, or even less frequently if bias is present. Epidemiological studies of an exploratory nature perform even worse, especially when underpowered, but even well-powered epidemiological studies may have only a one in five chance being true, if R = 1:10. Finally, in discovery-oriented research with massive testing, where tested relationships exceed true ones 1,000-fold (e.g., 30,000 genes tested, of which 30 may be the true culprits) [30,31], PPV for each claimed relationship is extremely low, even with considerable standardization of laboratory and statistical methods, outcomes, and reporting thereof to minimize bias.
Table 4
PPV of Research Findings for Various Combinations of Power (1 – ß), Ratio of True to Not-True Relationships (R), and Bias (u)
Claimed Research Findings May Often Be Simply Accurate Measures of the Prevailing Bias
As shown, the majority of modern biomedical research is operating in areas with very low pre- and post-study probability for true findings. Let us suppose that in a research field there are no true findings at all to be discovered. History of science teaches us that scientific endeavor has often in the past wasted effort in fields with absolutely no yield of true scientific information, at least based on our current understanding. In such a “null field,” one would ideally expect all observed effect sizes to vary by chance around the null in the absence of bias. The extent that observed findings deviate from what is expected by chance alone would be simply a pure measure of the prevailing bias.
For example, let us suppose that no nutrients or dietary patterns are actually important determinants for the risk of developing a specific tumor. Let us also suppose that the scientific literature has examined 60 nutrients and claims all of them to be related to the risk of developing this tumor with relative risks in the range of 1.2 to 1.4 for the comparison of the upper to lower intake tertiles. Then the claimed effect sizes are simply measuring nothing else but the net bias that has been involved in the generation of this scientific literature. Claimed effect sizes are in fact the most accurate estimates of the net bias. It even follows that between “null fields,” the fields that claim stronger effects (often with accompanying claims of medical or public health importance) are simply those that have sustained the worst biases.
For fields with very low PPV, the few true relationships would not distort this overall picture much. Even if a few relationships are true, the shape of the distribution of the observed effects would still yield a clear measure of the biases involved in the field. This concept totally reverses the way we view scientific results. Traditionally, investigators have viewed large and highly significant effects with excitement, as signs of important discoveries. Too large and too highly significant effects may actually be more likely to be signs of large bias in most fields of modern research. They should lead investigators to careful critical thinking about what might have gone wrong with their data, analyses, and results.
Of course, investigators working in any field are likely to resist accepting that the whole field in which they have spent their careers is a “null field.” However, other lines of evidence, or advances in technology and experimentation, may lead eventually to the dismantling of a scientific field. Obtaining measures of the net bias in one field may also be useful for obtaining insight into what might be the range of bias operating in other fields where similar analytical methods, technologies, and conflicts may be operating.
How Can We Improve the Situation?
Is it unavoidable that most research findings are false, or can we improve the situation? A major problem is that it is impossible to know with 100% certainty what the truth is in any research question. In this regard, the pure “gold” standard is unattainable. However, there are several approaches to improve the post-study probability.
Better powered evidence, e.g., large studies or low-bias meta-analyses, may help, as it comes closer to the unknown “gold” standard. However, large studies may still have biases and these should be acknowledged and avoided. Moreover, large-scale evidence is impossible to obtain for all of the millions and trillions of research questions posed in current research. Large-scale evidence should be targeted for research questions where the pre-study probability is already considerably high, so that a significant research finding will lead to a post-test probability that would be considered quite definitive. Large-scale evidence is also particularly indicated when it can test major concepts rather than narrow, specific questions. A negative finding can then refute not only a specific proposed claim, but a whole field or considerable portion thereof. Selecting the performance of large-scale studies based on narrow-minded criteria, such as the marketing promotion of a specific drug, is largely wasted research. Moreover, one should be cautious that extremely large studies may be more likely to find a formally statistical significant difference for a trivial effect that is not really meaningfully different from the null [32–34].
Second, most research questions are addressed by many teams, and it is misleading to emphasize the statistically significant findings of any single team. What matters is the totality of the evidence. Diminishing bias through enhanced research standards and curtailing of prejudices may also help. However, this may require a change in scientific mentality that might be difficult to achieve. In some research designs, efforts may also be more successful with upfront registration of studies, e.g., randomized trials [35]. Registration would pose a challenge for hypothesis-generating research. Some kind of registration or networking of data collections or investigators within fields may be more feasible than registration of each and every hypothesis-generating experiment. Regardless, even if we do not see a great deal of progress with registration of studies in other fields, the principles of developing and adhering to a protocol could be more widely borrowed from randomized controlled trials.
Finally, instead of chasing statistical significance, we should improve our understanding of the range of R values—the pre-study odds—where research efforts operate [10]. Before running an experiment, investigators should consider what they believe the chances are that they are testing a true rather than a non-true relationship. Speculated high R values may sometimes then be ascertained. As described above, whenever ethically acceptable, large studies with minimal bias should be performed on research findings that are considered relatively established, to see how often they are indeed confirmed. I suspect several established “classics” will fail the test [36].
Nevertheless, most new discoveries will continue to stem from hypothesis-generating research with low or very low pre-study odds. We should then acknowledge that statistical significance testing in the report of a single study gives only a partial picture, without knowing how much testing has been done outside the report and in the relevant field at large. Despite a large statistical literature for multiple testing corrections [37], usually it is impossible to decipher how much data dredging by the reporting authors or other research teams has preceded a reported research finding. Even if determining this were feasible, this would not inform us about the pre-study odds. Thus, it is unavoidable that one should make approximate assumptions on how many relationships are expected to be true among those probed across the relevant research fields and research designs. The wider field may yield some guidance for estimating this probability for the isolated research project. Experiences from biases detected in other neighboring fields would also be useful to draw upon. Even though these assumptions would be considerably subjective, they would still be very useful in interpreting research claims and putting them in context.
Abbreviation
PPV |
positive predictive value |
Footnotes
Citation: Ioannidis JPA (2005) Why most published research findings are false. PLoS Med 2(8): e124.
References
- Ioannidis JP, Haidich AB, Lau J. Any casualties in the clash of randomised and observational evidence? BMJ. 2001;322:879–880. [PMC free article] [PubMed] [Google Scholar]
- Lawlor DA, Davey Smith G, Kundu D, Bruckdorfer KR, Ebrahim S. Those confounded vitamins: What can we learn from the differences between observational versus randomised trial evidence? Lancet. 2004;363:1724–1727. [PubMed] [Google Scholar]
- Vandenbroucke JP. When are observational studies as credible as randomised trials? Lancet. 2004;363:1728–1731. [PubMed] [Google Scholar]
- Michiels S, Koscielny S, Hill C. Prediction of cancer outcome with microarrays: A multiple random validation strategy. Lancet. 2005;365:488–492. [PubMed] [Google Scholar]
- Ioannidis JPA, Ntzani EE, Trikalinos TA, Contopoulos-Ioannidis DG. Replication validity of genetic association studies. Nat Genet. 2001;29:306–309. [PubMed] [Google Scholar]
- Colhoun HM, McKeigue PM, Davey Smith G. Problems of reporting genetic associations with complex outcomes. Lancet. 2003;361:865–872. [PubMed] [Google Scholar]
- Ioannidis JP. Genetic associations: False or true? Trends Mol Med. 2003;9:135–138. [PubMed] [Google Scholar]
- Ioannidis JPA. Microarrays and molecular research: Noise discovery? Lancet. 2005;365:454–455. [PubMed] [Google Scholar]
- Sterne JA, Davey Smith G. Sifting the evidence—What’s wrong with significance tests. BMJ. 2001;322:226–231. [PMC free article] [PubMed] [Google Scholar]
- Wacholder S, Chanock S, Garcia-Closas M, Elghormli L, Rothman N. Assessing the probability that a positive report is false: An approach for molecular epidemiology studies. J Natl Cancer Inst. 2004;96:434–442. [PMC free article] [PubMed] [Google Scholar]
- Risch NJ. Searching for genetic determinants in the new millennium. Nature. 2000;405:847–856. [PubMed] [Google Scholar]
- Kelsey JL, Whittemore AS, Evans AS, Thompson WD. Methods in observational epidemiology, 2nd ed. New York: Oxford U Press; 1996. 432 pp. [Google Scholar]
- Topol EJ. Failing the public health—Rofecoxib, Merck, and the FDA. N Engl J Med. 2004;351:1707–1709. [PubMed] [Google Scholar]
- Yusuf S, Collins R, Peto R. Why do we need some large, simple randomized trials? Stat Med. 1984;3:409–422. [PubMed] [Google Scholar]
- Altman DG, Royston P. What do we mean by validating a prognostic model? Stat Med. 2000;19:453–473. [PubMed] [Google Scholar]
- Taubes G. Epidemiology faces its limits. Science. 1995;269:164–169. [PubMed] [Google Scholar]
- Golub TR, Slonim DK, Tamayo P, Huard C, Gaasenbeek M, et al. Molecular classification of cancer: Class discovery and class prediction by gene expression monitoring. Science. 1999;286:531–537. [PubMed] [Google Scholar]
- Moher D, Schulz KF, Altman DG. The CONSORT statement: Revised recommendations for improving the quality of reports of parallel-group randomised trials. Lancet. 2001;357:1191–1194. [PubMed] [Google Scholar]
- Ioannidis JP, Evans SJ, Gotzsche PC, O’Neill RT, Altman DG, et al. Better reporting of harms in randomized trials: An extension of the CONSORT statement. Ann Intern Med. 2004;141:781–788. [PubMed] [Google Scholar]
- International Conference on Harmonisation E9 Expert Working Group. ICH Harmonised Tripartite Guideline. Statistical principles for clinical trials. Stat Med. 1999;18:1905–1942. [PubMed] [Google Scholar]
- Moher D, Cook DJ, Eastwood S, Olkin I, Rennie D, et al. Improving the quality of reports of meta-analyses of randomised controlled trials: The QUOROM statement. Quality of Reporting of Meta-analyses. Lancet. 1999;354:1896–1900. [PubMed] [Google Scholar]
- Stroup DF, Berlin JA, Morton SC, Olkin I, Williamson GD, et al. Meta-analysis of observational studies in epidemiology: A proposal for reporting. Meta-analysis of Observational Studies in Epidemiology (MOOSE) group. JAMA. 2000;283:2008–2012. [PubMed] [Google Scholar]
- Marshall M, Lockwood A, Bradley C, Adams C, Joy C, et al. Unpublished rating scales: A major source of bias in randomised controlled trials of treatments for schizophrenia. Br J Psychiatry. 2000;176:249–252. [PubMed] [Google Scholar]
- Altman DG, Goodman SN. Transfer of technology from statistical journals to the biomedical literature. Past trends and future predictions. JAMA. 1994;272:129–132. [PubMed] [Google Scholar]
- Chan AW, Hrobjartsson A, Haahr MT, Gotzsche PC, Altman DG. Empirical evidence for selective reporting of outcomes in randomized trials: Comparison of protocols to published articles. JAMA. 2004;291:2457–2465. [PubMed] [Google Scholar]
- Krimsky S, Rothenberg LS, Stott P, Kyle G. Scientific journals and their authors’ financial interests: A pilot study. Psychother Psychosom. 1998;67:194–201. [PubMed] [Google Scholar]
- Papanikolaou GN, Baltogianni MS, Contopoulos-Ioannidis DG, Haidich AB, Giannakakis IA, et al. Reporting of conflicts of interest in guidelines of preventive and therapeutic interventions. BMC Med Res Methodol. 2001;1:3. [PMC free article] [PubMed] [Google Scholar]
- Antman EM, Lau J, Kupelnick B, Mosteller F, Chalmers TC. A comparison of results of meta-analyses of randomized control trials and recommendations of clinical experts. Treatments for myocardial infarction. JAMA. 1992;268:240–248. [PubMed] [Google Scholar]
- Ioannidis JP, Trikalinos TA. Early extreme contradictory estimates may appear in published research: The Proteus phenomenon in molecular genetics research and randomized trials. J Clin Epidemiol. 2005;58:543–549. [PubMed] [Google Scholar]
- Ntzani EE, Ioannidis JP. Predictive ability of DNA microarrays for cancer outcomes and correlates: An empirical assessment. Lancet. 2003;362:1439–1444. [PubMed] [Google Scholar]
- Ransohoff DF. Rules of evidence for cancer molecular-marker discovery and validation. Nat Rev Cancer. 2004;4:309–314. [PubMed] [Google Scholar]
- Lindley DV. A statistical paradox. Biometrika. 1957;44:187–192. [Google Scholar]
- Bartlett MS. A comment on D.V. Lindley’s statistical paradox. Biometrika. 1957;44:533–534. [Google Scholar]
- Senn SJ. Two cheers for P-values. J Epidemiol Biostat. 2001;6:193–204. [PubMed] [Google Scholar]
- De Angelis C, Drazen JM, Frizelle FA, Haug C, Hoey J, et al. Clinical trial registration: A statement from the International Committee of Medical Journal Editors. N Engl J Med. 2004;351:1250–1251. [PubMed] [Google Scholar]
- Ioannidis JPA. Contradicted and initially stronger effects in highly cited clinical research. JAMA. 2005;294:218–228. [PubMed] [Google Scholar]
- Hsueh HM, Chen JJ, Kodell RL. Comparison of methods for estimating the number of true null hypotheses in multiplicity testing. J Biopharm Stat. 2003;13:675–689. [PubMed] [Google Scholar]
Fra Wikipedia, den frie encyklopedi
Hopp til navigeringHopp til søk

Astrologi eller stjernetydning er en flere tusen år gammel pseudovitenskapelig metode og overtro for å forutsi framtida og få kunnskap om menneskelivet. Bildet viser den estiske spåmannen Igor Mang (født 1949) og en plansje med stjernetegnene i zodiaken.
Foto: Maria Laanjärv, 2014
Pseudovitenskap (fra gresk ψεύδω pséudō- «falsk») er noe som virker som eller utgir seg for å være vitenskapelig, men som ikke følger alminnelig anerkjente kriterier for å regnes som vitenskap eller ikke har status som vitenskap.[1] Begrepet benyttes oftest nedsettende[2] og få benytter begrepet pseudovitenskap om egne oppfatninger. Vitenskapelig utdanning innebærer blant annet opplæring i å skjelne vitenskapelige fakta og teorier fra pseudovitenskap.[3]
Astrologi, alkymi og Myers-Briggs personlighetstest er eksempler på pseudovitenskap.[4][5][6][7] Homøopati og akupunktur er eksempler på alternativ behandling som bygger på pseudovitenskap.[8]
Pseudovitenskap kan vanskelig defineres klart og entydig. Denne utfordringen med å fastsette kriterier som skjelner mellom vitenskap og pseudovitenskap, den såkalte demarkasjonen, kalles demarkasjonsproblemet etter Karl Popper.[1][9][10] Popper understreket at skillet mellom vitenskap og pseudovitenskap ikke er det samme som skillet mellom sant og usant: Vitenskap kan omfatte noe som er usant og pseudovitenskap kan slumpe til å inneholde noe som viser seg å være sant.[11]
Pseudovitenskap deler en del trekk med vitenskap. Forskere innenfor pseudovitenskap har ambisjoner om eller gir seg ut for å drive vitenskapelig arbeid.[9] Pseudovitenskap gir overfladisk inntrykk av å være vitenskapelig ved bruk av faglig sjargong for å fremstille vidtrekkende og imponerende teorier. Utøvere av pseudovitenskap hevder at teoriene er godt støttet av fakta og kritikk møtes av sofistikerte argument. En grunnleggende forskjell er at pseudovitenskap er statisk og ikke forkaster eller endrer sine teorier på bakgrunn av fakta slik normal vitenskap gjør.[9][12] Vitenskap kjennetegnes ved forskernes arbeidsform og metode, ikke ved innholdet i forskningen eller teoriene.[10] I noen tilfeller tas kritikk av forskningen som bevis på at teorien, noe som forekommer blant annet i Freuds psykoanalyse og blant forskere inspirert av marxisme.[2][13] Imre Lakatos mente forskningsprogrammer består av en hard kjerne som til en viss grad er beskyttet mot falsifisering. Et forskningsprogram er “progressivt” dersom teoriene gjør nye prediksjoner og nye oppdagelser, ellers er programmet i ferd med å “degenere” og bli pseudovitenskapelig.[2] Derksen argumenterer for at det først og fremst dreier seg om pseudoforskere eller -forskning, ikke nødvendigvis et helt forskningsfelt.[9]
Betegnelsen pseudovitenskap har vært brukt om ideer eller virksomhet som de aller fleste i det minste med tilbakeblikk oppfatter som bisarre, forfeilet eller ubrukelige, men som kan ha mange tilhengere i en periode. Bruk av merkelappen pseudovitenskap er stort sett lite kontroversielt i slike tilfeller. En annen bruk av betegnelsen handler om grensetrekning (demarkasjon) mellom seriøs vitenskap og annen virksomhet. Denne andre bruken av betegnelsen knytter seg til mer avanserte faglige eller filosofiske debatter. Merkelappen pseudovitenskap på frenologi var opprinnelig kontroversielt, men frenologi anses nå som bisarr og forfeilet som vitenskap.[2]
Bunge skjelner mellom forestillingsfelt («belief fields)» og forskningsfelt («research fields»), der forestillingsfelt inkluderer religion, politiske ideologier og pseudovitenskap. Ifølge Bunge er et typisk for pseudovitenskap at det består av et fellesskap av «troende» som kaller seg forskere uten drive forskning i henhold til vitenskapelig standarder. Pseudovitenskap blir holdt i live blant annet av kommersielle interesser. Bunge skriver at pseudovitenskap baserer seg på en filosofi som tillater uobserverbare størrelser, dogmer og generell mangel på klarhet; pseudovitenskaper baserer seg ofte på ikke-testbare hypoteser eller hypoteser som er i strid med veletablert kunnskap.[14]
Følgende egenskaper nevnes som typiske:
- Påstandene er ikke falsifiserbare,[4][12] ifølge Popper er falsifiserbarhet det avgjørende kriteriet for å skjelne vitenskap fra pseudovitenskapelig og ikke-vitenskapelig virksomhet.[10] Popper fremholdt at induktiv metode ikke skiller vitenskap og pseudovitenskap.[11]
- Vaghet, mangel på klare definisjoner, ekstrem mangel på presisjon[12][14] som gjør at påstander vanskelig kan etterprøves gjennom målinger og tester. Påstander lar seg ikke bekrefte.[4]
- Arbeidet søker ikke den forklaringen som krever færrest mulig antakelser (Ockhams barberkniv-prinsipp).
- Motstand mot vitenskapelig testing fra personen eller miljøet som legger fram arbeidet;[12] angrep på kritikere.[15]
- Fagmiljøet driver ikke systematisk og kritisk prøving av sine teorier, og vurderer ikke egne teorier mot alternative teorier.[4][12]
- Mangel på dokumentasjon;[9] selektiv bruk/tolkning av bevismateriale,[9] overdreven vekt på anekdotisk bevisføring og «spektakulære sammentreff».[9]
- Omvendt bevisbyrde (det at en udokumentert påstand ikke lar seg motbevise tas som bekreftelse på at den er sann).
- Liten grad av utvikling, endring og progresjon;[4][9][14] dogmatisk og til dels paranoid overfor annen forskning.[12]
- Feilaktig eller misvisende bruk av vitenskapelig terminologi.
- Tendens til å isolere seg fra andre deler av forskningsmiljøet;[12][12][14] truende insinuasjoner mot mainstream forskning og kritikere.[16]
- Bruk av forsvarsmekanismer eller «immuniseringsstrategier» for å beskytte egen teori/forskning mot kritikk.[9][13]
- Altomfattende teorier og overdrevne forestillinger om betydningen av egen teori; overdreven generalisering fra empiri.[9]
Vitenskapsfilosofen Paul Feyerabend argumenterte for at et skille mellom vitenskap og ikke-vitenskap hverken er mulig eller ønskelig.[17] [note 1].
Forhold som kan vanskeliggjøre et skille er variabelt tempo i utvikling av vitenskapelige teorier og metoder i lys av nye data.[klargjør][note 2].
Larry Laudan mente at «pseudovitenskap» ikke har en vitenskapelig betydning og brukes for det meste for å beskrive følelser. Laudan skrev at dersom vi ønsker å reise oss og stå på fornuftens side burde vi sløyfe begreper som «pseudovitenskap» og «uvitenskapelig» fra vårt vokabular, og at de bare er hule fraser som utelukkende har emosjonell funksjon.[18] Richard McNally hevdet at begrepet pseudovitenskap har blitt lite mer enn et krenkende moteord for raskt å avvise [synspunkter] hos meningsmotstandere i raske media lyd-klipp. Videre skrev han at når terapeutiske foregangspersoner fremmer påstander om sine intervensjoner, skal vi ikke kaste bort tiden med å granske om intervensjonene er pseudovitenskapelige. Ifølge McNally bør man heller spørre: “Hvordan vet du at din intervensjon virker? Hva er dine bevis?”.[19]
Pseudovitenskap forveksles gjerne med kvasivitenskap, som er aktivitet som har bare noen av kjennetegnene som forventes til seriøs vitenskap.[20] Ikke-falsifiserbare teorier er ikke nødvendigvis pseudovitenskapelige, de kan i stedet være metafysiske eller ikke-vitenskapelige.[9]
Historisk anvendelse av begrepet pseudovitenskap[rediger | rediger kilde]
Den første kjent bruken av «pseudovitenskap» er fra 1797 da historikeren James Pettit Andrew omtalte alkemi som en «fantastical pseudo-science». Betegnelsen har vært jevnlig brukt siden 1880 og har hatt en klart nedsettende betydning.[1][21][22] Karl Popper mente at psykoanalyse er pseudovitenskap fordi den ikke er falsifiserbar.[23] Popper mente også at marxistisk teori er pseudovitenskapelig.[24] Frenologi ble tidlig på 1800-tallet beskrevet som pseudovitenskap.[2]
Overganger mellom pseudovitenskap og vitenskap[rediger | rediger kilde]
Enkelte historiske pseudovitenskaper, som alkymi og astrologi[4][5], kan regnes som forløpere for nåværende vitenskaper.[trenger referanse] Disse fagene kan da først betegnes som pseudovitenskapelige når noen fastholder at disse er sanne på tross av at teoriene var erstattet med nye forklaringer som passer bedre til observasjonene. Alternativ behandling har også i stor grad manglende vitenskapelig grunnlag,[25][26] og behandlingsformer som forklarer behandlingens virkning ved hjelp av forklaringer som er i strid med vitenskap betegnes som pseudovitenskap.[27] Hvordan skillelinjen trekkes mellom vitenskap og pseudovitenskap har innvirkning på forståelsen av medisin og forståelsen av enkelte religiøse oppfatninger.[trenger referanse]
Flere disipliner som i dag betegnes som pseudovitenskap, har tidligere blitt betraktet som seriøs vitenskap – i noen tilfeller langt ut på 1900-tallet (for eksempel rasebiologi). Det finnes også eksempler på det motsatte: at arbeider som tidligere har vært ansett som pseudovitenskapelige i ettertid har fått status som vitenskap, for eksempel hypotesen om kontinentaldrift.
Astrologi og alkymi er eksempler på pseudovitenskap.[4][5] Flere medisinske behandlingsformer bygger på pseudovitenskap blant annet homøopati og akupunktur.[8] Freuds empiriske grunnlag for psykoanalyse regnes som pseudovitenskapelig.[9] Christian Science beskrives som pseudovitenskap.[9] Nazistene legitimerte folkemord med pseudovitenskap om raser og gener, skriver Steven Pinker.[28] Eye movement desensitization and reprocessing, en form for terapi mot post-traumatisk stress, har blitt karakterisert som pseudovitenskapelig.[2] Karl Marx historieforskning har blitt karakterisert som pseudovitenskapelig blant annet av Karl Popper[29] og Imre Lakatos. Trofim Lysenkos landbruksforskning ble på samme måte som raseteorien i Hitler-Tyskland den offisielle læren fastsatt av myndighetene.[2][30] Shermer anser antroposofisk medisin og biorytme som pseudovitenskapelig.[16] Alternativ medisin og relatert funksjonell ernæring regnes som pseudovitenskap.[25][31][32]
Tilhengere av pseudovitenskap holder fast på eldgamle tanker, slik som at stjerner og planeter påvirker en persons indre sjelsliv, eller at tidligere antatte medisiner som ikke har dokumentert effekt likevel fungerer. Tilhengere av pseudovitenskapelige teorier motsetter seg vanligvis å få sin tro omtalt som pseudovitenskap, siden begrepet oppfattes som nedsettende.
Isaac Newton var en ivrig alkymist, og ideen om at usynlig kraft styrte jordens bane rundt solen ble i samtiden ansett som uvitenskapelig.[33]
- ^ ‘A particularly radical reinterpretation of science comes from Paul Feyerabend, “the worst enemy of science”… Like Lakatos, Feyerabend was also a student under Popper. In an interview with Feyerabend in Science, [he says] “Equal weight… should be given to competing avenues of knowledge such as astrology, acupunture, and witchcraft…”‘[trenger referanse]
- ^ “We can now propose the following principle of demarcation: A theory or discipline which purports to be scientific is pseudoscientific if and only if: it has been less progressive than alternative theories over a long period of time, and faces many unsolved problems; but the community of practitioners makes little attempt to develop the theory towards solutions of the problems, shows no concern for attempts to evaluate the theory in relation to others, and is selective in considering confirmations and non confirmations.” Thagard, Paul R. (1978). «Why Astrology is a Pseudoscience». PSA: Proceedings of the Biennial Meeting of the Philosophy of Science Association. 1978: 223–234. Besøkt 5. september 2018.
- ^ a b c Hansson, Sven Ove (2017). «Science and Pseudo-Science». I Zalta, Edward N. The Stanford Encyclopedia of Philosophy. Metaphysics Research Lab, Stanford University. Besøkt 13. august 2017.
- ^ a b c d e f g Still, A., & Dryden, W. (2004). The social psychology of “pseudoscience”: A brief history. Journal for the theory of social behaviour, 34(3), 265-290.
- ^ Hurd, P. D. (1998). Scientific literacy: New minds for a changing world. Science education, 82(3), 407-416.
- ^ a b c d e f g Thagard, P. R. (1978, January). Why astrology is a pseudoscience. In PSA: Proceedings of the Biennial Meeting of the Philosophy of Science Association (Vol. 1978, No. 1, pp. 223-234). Philosophy of Science Association.
- ^ a b c Allchin, D. (2004). Pseudohistory and pseudoscience. Science & Education, 13(3), 179-195.
- ^ Chen, Angus. «How Accurate Are Personality Tests?». Scientific American (engelsk). Besøkt 22. august 2022.
- ^ Stromberg, Joseph (15. juli 2014). «Why the Myers-Briggs test is totally meaningless». Vox (engelsk). Besøkt 22. august 2022.
- ^ a b Stalker, D., & Glymour, C. (1985). Examining Holistic Medicine. Prometheus Books, Buffalo NY.
- ^ a b c d e f g h i j k l m Derksen AA (1993). «The seven sins of pseudo-science». Journal of General Philosophy of Science. 24: 17–42. doi:10.1007/BF00769513.[død lenke]
- ^ a b c Shermer, Michael (1. september 2011). «What Is Pseudoscience?». Scientific American (engelsk). Besøkt 13. august 2017.
- ^ a b Fjelland, Ragnar (1999). Innføring i vitenskapsteori. Oslo: Universitetsforl. ISBN 8200129772.
- ^ a b c d e f g h Martin, M. (1994). Pseudoscience, the paranormal, and science education. Science & Education, 3(4), 357-371.
- ^ a b Boudry, M., & Braeckman, J. (2011). Immunizing strategies and epistemic defense mechanisms. Philosophia, 39(1), 145-161.
- ^ a b c d Bunge, M. (1984). What is pseudoscience. The Skeptical Inquirer, 9(1), 36-47.
- ^ Pratkanis, Anthony R (Juli–august 1995). «How to Sell a Pseudoscience». Skeptical Inquirer. 19 (4): 19–25.
- ^ a b Shermer, M. (2002). The Skeptic Encyclopedia of Pseudoscience. ABC-CLIO.
- ^ Feyerabend, P. (1975) Against Method: Outline of an Anarchistic Theory of Knowledge ISBN 0-86091-646-4 Table of contents and final chapter.
- ^ Laudan, L. (1996) “The demise of the demarcation problem” in Ruse, Michael, But Is It Science?: The Philosophical Question in the Creation/Evolution Controversy pp. 337–350.
- ^ McNally RJ (2003) Is the pseudoscience concept useful for clinical psychology? The Scientific Review of Mental Health Practice, vol. 2, no. 2 (Fall/Winter 2003)
- ^ Bernie Garrett (27. april 2012). «Non-science, pseudoscience, quasi-science and bad science; is there a difference?». Roger & Bernies Real Science Blog (engelsk). Besøkt 13. august 2017.
- ^ http://ieg-ego.eu/en/threads/crossroads/knowledge-spaces/ute-frietsch-the-boundaries-of-science-pseudoscience
- ^ Frietsch, Ute (7. april 2015). «The Boundaries of Science / Pseudoscience». European History Online.
- ^ Grünbaum, A. (1979). Is Freudian psychoanalytic theory pseudo-scientific by Karl Popper’s criterion of demarcation?. American Philosophical Quarterly, 16(2), 131-141.
- ^ Hudelson, R. (1983). A reply to Farr. Philosophy of the Social Sciences, 13(4), 473.
- ^ a b Sampson, W. (1995). ANTISCIENCE TRENDS IN THE RISE OF THE “ALTERNATIVE MEDICINE’MOVEMENT. Annals of the New York Academy of Sciences, 775(1), 188-197.
- ^ Angell, M., and Jerome P. Kassirer: Alternative Medicine — The Risks of Untested and Unregulated Remedies New England Journal of Medicine september 1998; 339:839-841
- ^ The Dangers of Pseudoscience New York Times
- ^ Pinker, Steven (7. januar 2009). «My Genome, My Self – Steven Pinker Gets to the Bottom of his own Genetic Code». The New York Times (engelsk). ISSN 0362-4331. Besøkt 15. august 2017.
- ^ Hudelson, R. (1980). Popper’s critique of Marx. Philosophical Studies, 37(3), 259-270.
- ^ Roll-Hansen, Nils (1985). Ønsketenkning som vitenskap: Lysenkos innmarsj i sovjetisk biologi 1927-37. Oslo: Universitetsforlaget. ISBN 8200074390.
- ^ Beyerstein, B. (1997). Alternative medicine: Where’s the evidence?. Can J Public Health, 88(3), 149-50.
- ^ Durant, J. R. (1998). Alternative medicine: an attractive nuisance. Journal of clinical oncology, 16(1), 1-2.
- ^ Was Newton a scientist or a sorcerer?
- Derksen AA (1993). «The seven sins of pseudo-science». Journal of General Philosophy of Science. 24: 17–42. doi:10.1007/BF00769513.[død lenke]
- Derksen A. A. (2001). «The seven strategies of the sophisticated pseudo-scientist: a look into Freud’s rhetorical toolbox». J Gen Phil Sci. 32 (2): 329–350. doi:10.1023/A:1013100717113.
- Pratkanis, Anthony R (Juli–august 1995). «How to Sell a Pseudoscience». Skeptical Inquirer. 19 (4): 19–25.
- Shermer, M. (2002). Why people believe weird things: Pseudoscience, superstition, and other confusions of our time. Holt Paperbacks. ISBN 0805070893
- Shermer, M. (2002). The Skeptic Encyclopedia of Pseudoscience. ABC-CLIO.
BMC Pediatrics https://bmcpediatr.biomedcentral.com/track/pdf/10.1186/1471-2431-14-88.pdf
Yi Ji1*, Siyuan Chen1,2, Lin Zhong1
, Xiaoping Jiang1
, Shuguang Jin1
, Feiteng Kong1
, Qi Wang1
, Caihong Li3
and Bo Xiang1
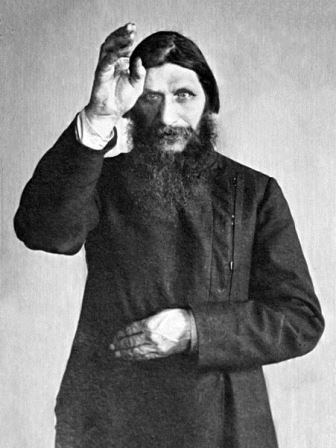
Image of Rasputin Courtesy of Wikimedia commons
Grigori Yefimovich Rasputin was murdered on this day 100 years ago in Petrograd. The date in the Russian Julian calendar was 17 December, but for the Western, Gregorian calendar it was 30 December.
The lurid details of his murder are well-known, mainly due to the memoirs his murderers wrote about the act. The most famous account was written by Prince Felix Yusupov.
Why was Rasputin so hated?
Rasputin had risen to immense power from humble beginnings. He was born in 1869 in Pokrovskoe, Siberia. In 1892 he had a religious calling and left his family to wander through Russia, seeking spiritual enlightenment. He became known as a ‘holy man’ and drew followers to him. In 1905 he met the royal family and soon became part of their inner circle. He seemed to be able to stop the bleeding of their haemophiliac son, Alexei. How he did this is still not fully understood.
However, Rasputin made enemies because he meddled in politics and got ministers he disliked dismissed. I found evidence of the role he played in getting ‘Monsieur de Sazonow’ sacked from his post as Foreign Minister and the unpopular dismissal of the Minister Samarin, both in FO 800/75. Rasputin was often described as a conman who was a promiscuous drunkard. In FO 800/178, a memo by Lord Bertie mentions how Prince Orlof tried to explain to the Tsar the danger of the royal family being connected to such a dissolute character and of Rasputin’s ‘boastings’ about being the lover of the Tsarina. The Tsar reacted furiously and refused to receive Prince Orlof again.
Rasputin’s murderers believed they were saving Russia from his baleful influence. The plot involved Prince Felix Yusupov, the Grand Duke Dmitry Pavlovich, Lieutenant Sergei Sukhotin, Vladimir Purishkevich and the doctor Stanislaus Lazovert.
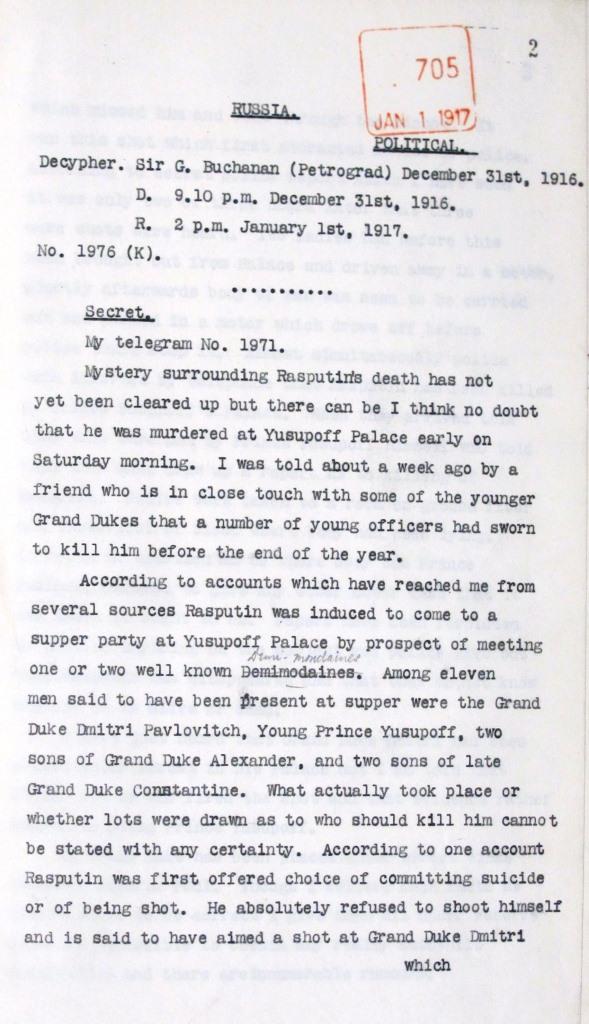
Rasputin was lured to Yusupov Palace (catalogue reference: FO 371/2994 (705))
According to Prince Felix’s account, Rasputin was driven to Yusupovs palace on the Moika after midnight on 30 December. In the document FO 371/2994 it clearly states that Rasputin was lured to the palace.
Rasputin went down to the cellar with Yusupov and allegedly ate cakes and Madeira wine laced with poison but suffered no ill-effects. At around 02:30, Yusupov was getting worried. He ran upstairs to his fellow conspirators to tell them the poison was not working. Yusupov grabbed a gun and went down and shot Rasputin in the side. They all thought he was dead.
Pavlovich, Sukhotin and Lazovert then drove back to Rasputin’s home with Sukhotin dressing up in Rasputin’s overcoat and hat to make it look like he came home. Meanwhile Purishkevich and Yusupov stayed at the palace. Again, according to Yusupov when he returned to the cellar Rasputin opened his eyes and screamed. He jumped to his feet and began to attack Yusupov. In each retelling of this story Yusupov made Rasputin more superhuman and demonic, probably as Douglas Smith argues in Rasputin to assuage his guilt at killing an unarmed man.
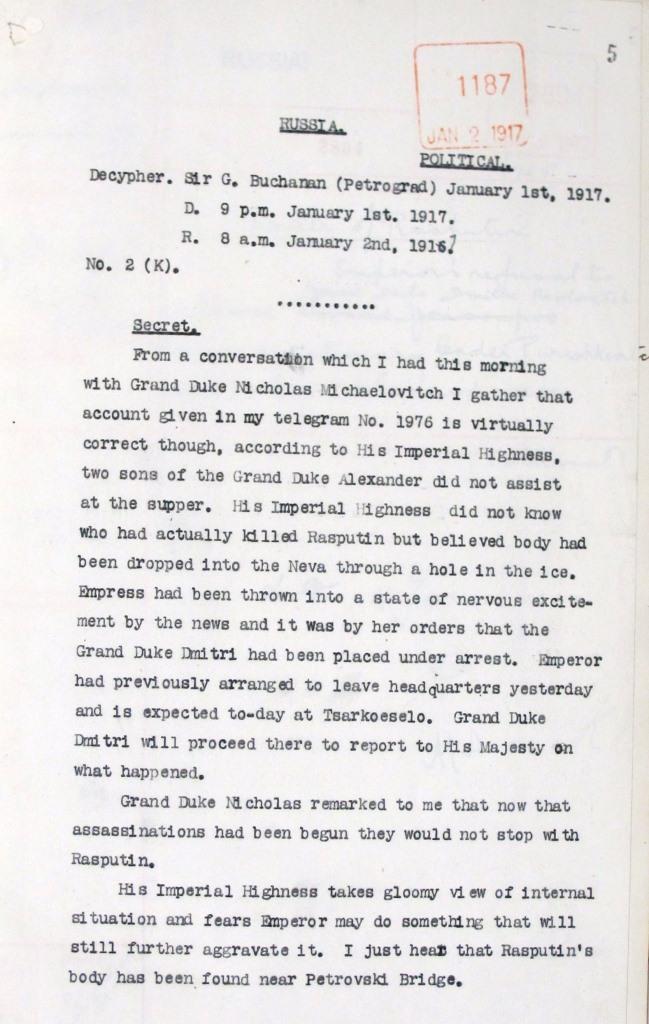
Rasputin’s body found near Petrovski Bridge (catalogue reference: FO 371/2994 (1187))
Yusupov ran out of the cellar and Rasputin escaped out of a side door into the courtyard, crawling and screaming like a ‘wounded animal’. Purishkevich, according to his version of events, shot four times at Rasputin; two shots missed, the third hit him in the back and the fourth in the head. Others, such as the Tsar’s eldest daughter, Olga, believed that Grand Duke Dmitry, a professional soldier, fired the fatal shot.
The murderers tied up Rasputin’s body with ropes, wrapped it in some blue fabric, put it in the car and then drove to Petrovski bridge. His body was dropped into a hole in the ice of the river Neva. According to Rasputin’s daughter he was still alive when thrown into the water.
Rumours immediately spread that Rasputin had been murdered. There is evidence of this in the document FO 371/2741.
The Minister of the Interior Protopopov started an investigation into the disappearance and then murder of Rasputin. It was run by General Pyotr Popov. On the afternoon of 30 December a brown boot was retrieved from the frozen water; blood was also noticed on the railings of the bridge. The river police were called in and found Rasputin’s body in the icy river on 1 January (19 December in the Russian calendar). The document FO/371/2994 reiterates this chain of events – the belief that Rasputin’s body was dropped into the river Neva and the news that his body had just been found near Petrovski bridge.
The autopsy on the body took place around 22:00 of that day. It was conducted by Dr Dmitry Kosorotov. He found that Rasputin was shot three times. One shot was on the left side of his chest, one in his back and the fatal shot was in his forehead. Dr Kosorotov also found there were no traces of poison in Rasputin’s body. The doctor Lazovert later stated he had a pang of conscience and had put a harmless substance on the cakes, not powdered cyanide. The autopsy also proved that Rasputin had no water in his lungs and was dead before he was thrown into the water.
Many Russians, Swedes and Germans at the time believed that the British Secret Service had been involved in the murder. The motive was that Rasputin was known to be against the war and the British feared he would try to broker a peace deal with Germany.
According to Andrew Cook’s To kill Rasputin the British agent involved in the murder, possibly even firing the fatal shot, was Oswald Rayner, a close friend of Yusupov from his time at Oxford University. The Tsar himself said to the British Ambassador George Buchanan that he’d heard British agents were involved in the murder. The Ambassador strongly denied it. A document in our collection does show that George Buchanan had heard about a plot to kill Rasputin roughly a week before it happened (FO 371/2994).This shows Buchanan had prior knowledge of a plot but there is no evidence to suggest the British were involved. Unusually, his murderers were proud to proclaim what they had done and many Russians regarded them as patriotic heroes.
So what happened to Rasputin’s murderers? They literally got away with murder. Prince Yusupov was banished to a distant family estate and Grand Duke Dmitry was exiled to Persia. The others got no punishment and most of them slipped back into obscurity.
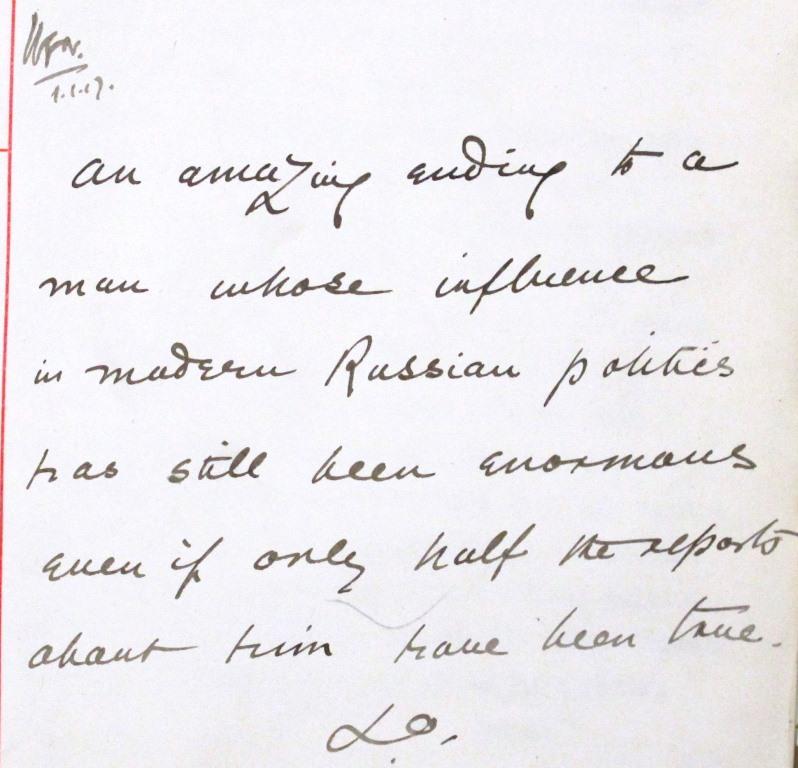
Memo mentioning ‘amazing ending’ and ‘enormous influence’ of Rasputin (catalogue reference: FO 371/2994(705))
Yusupov moved to America and, short of money, began cashing in on his notoriety as Rasputin’s murderer by writing several sets of memoirs about the event. He died in 1967.
To find out more about this subject I recommend the following books:
Det kalles et hjuldyr på norsk, bdelloid rotifers på latin. Nå har forskere funnet et 24.000 år gammelt hjuldyr under permafrosten i Sibir.